What we do
We are passionate about biodiversity and our mission is to combat the ongoing biodiversity crisis by providing and analyzing high-resolution biodiversity data. One focus is to improve field- and lab-work protocols to produce more accurate biodiversity assessments using environmental DNA. The other focus of our group is to develop computational methods that utilize these data to help us better understand and manage the spatial distribution of biodiversity.
Our research is interdisciplinary, including the fields of ecology, molecular biology, geomatics, bioinformatics, data science, and machine learning. If you have a background or interest in any of these fields and care about contributing with your work towards helping biodiversity, the Biodiversity Data Lab is the place for you!
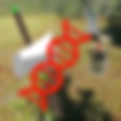
Biodiversity data
We are developing and applying genetic methods to detect small traces of DNA that organisms leave behind in their habitat (environmental DNA). This provides us with comprehensive biodiversity measures of the species community at a site, which even includes the large hidden diversity of species that have not yet been scientifically described (the vast majority of species!).
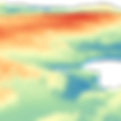
Spatial data
In our computational models we apply high-resolution geographic data produced by different remote sensing techniques. Some examples are satellite images or 3D-point clouds from airborne laser-scanning. We use these data to correlate biodiversity measures with the complex biotic and abiotic environment. Our aim is to eventually be able to make biodiversity predictions for areas that lack biodiversity data, allowing the generation of biodiversity heat maps.

Machine Learning
We are actively working on building state-of-the-art machine learning models to learn the complex associations of species with their environment. These models are capable to detect areas that are of high conservation value and can aid in conservation planning and selecting sites for biodiversity offsets. Further, these methods allow us to simulate the expected response of biodiversity to specific management scenarios or other factors such as climate change.